
Controlling ControlNet
Using a Latent Diffusion Model to Augment the Schematic Design Process
In collaboration with Joel Esposito
,
This research investigates how AI image generators can enhance architectural schematic design workflows. Control images (in this case, schematic or parti sketches) were paired with Stable Diffusion via ControlNet to produce output images that predictably reflect a specific design intent. Varying levels of detail (LOD) were established for both the schematic sketch and text prompts in order to examine the impact of visual and linguistic specificity on the final output. Understanding the synergistic relationship between these variables will help develop an intuitive understanding of how best to employ image generators as a tool in architectural design.
Workflow
A flow chart illustrating two potential workflows for incorporating AI image generation in the schematic design phase.
Parti Matrix Test
In an effort to understand the qualitative impact of the two primary inputs (the prompt and the control image) on the final output, an experiment was designed to compare varying combinations of image LOD and prompt LOD. Prompt LOD was arranged on the x-axis and Image LOD on the y-axis. The resultant chart enables a quick comparison of different combinations on both axes. Note how the level of inference achieved by the diffusion model becomes increasingly sophisticated, albeit in different domains, as each axis approaches a high LOD. For example, contextually-appropriate shading appears with the highest prompt specificity, while fine details like fenestration are more accurately rendered with a high detail input sketch. Due to the speed of output offered by the diffusion model, the number of generation cycles for each image was not controlled in this initial experiment — the additional marginal time involved was not considered of significance for business application in an architectural practice. Number of generation cycles will be tracked in any future testing.
Stroke Weight Testing
The apparent correlation between stroke weight and level of detail is noteworthy. Further testing will be required to determine the exact nature of how stroke weight interfaces with other input variables.
Chatbot Integration
Using Bing AI Chat (a large English language model based chatbot) to rapidly develop robust lists of architectural vocabulary for further experimentation.
Semantic Analysis
Future experiments can include leveraging other variables such as the semantic proximity of words within a given prompt.
Workflow “A” (Studio Work Example)
Example of workflow “A” used to rapidly develop spatial ideas, building massing, and context. Resultant sketches can be brought back into the diffusion model for rendering or reused in ControlNet. The feedback loop between designer and the diffusion model augments a priori expertise of the designer with the speed, fidelity, and breadth offered by the image generator.
Copyright Considerations
Artifacts from vast image data sets sometimes include agglomerated artist signatures. This raises important questions about the “opt-out only” nature of content that is utilized by image generators and the essential role that the data set plays in AI systems. The discourse surrounding copyright and image generation is rapidly evolving. At the present time, AI images are a catch-22 from a business perspective—they enable much faster workflows, but the raw output is not eligible for copyright. Direct manipulation of the output by the artist and/or more direct involvement of the artist during image creation (e.g. NVIDIA Canvas) will almost certainly be required to establish copyright over the image.
Looking Forward
The English language version of XKool AI Cloud is one example of a recently launched platform that offers a user-friendly interface as well as a robust feature set that can facilitate the aforementioned workflows with a lower bar to entry (faster learning curve).
This research investigated how diffusion based image generators paired with designer input can enhance traditional workflows. A variety of topics were explored ranging from understanding input variables to researching implications of copyright on image output. This project has set the foundation for further research into how architects and designers are applying image generators in their practice, and how architectural and intellectual copyright will impact the application of ai-based or ai-assisted designs.
Gallery
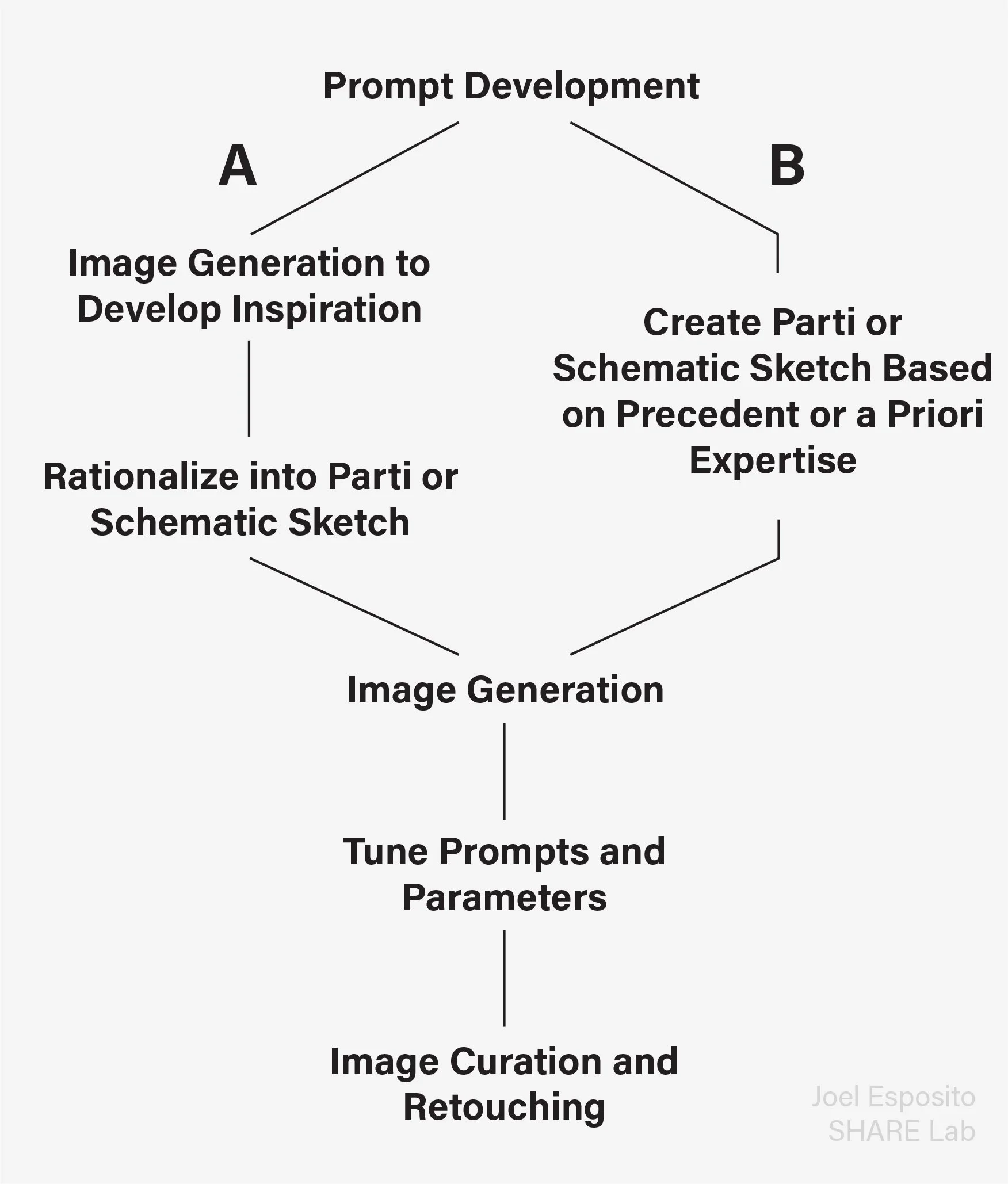
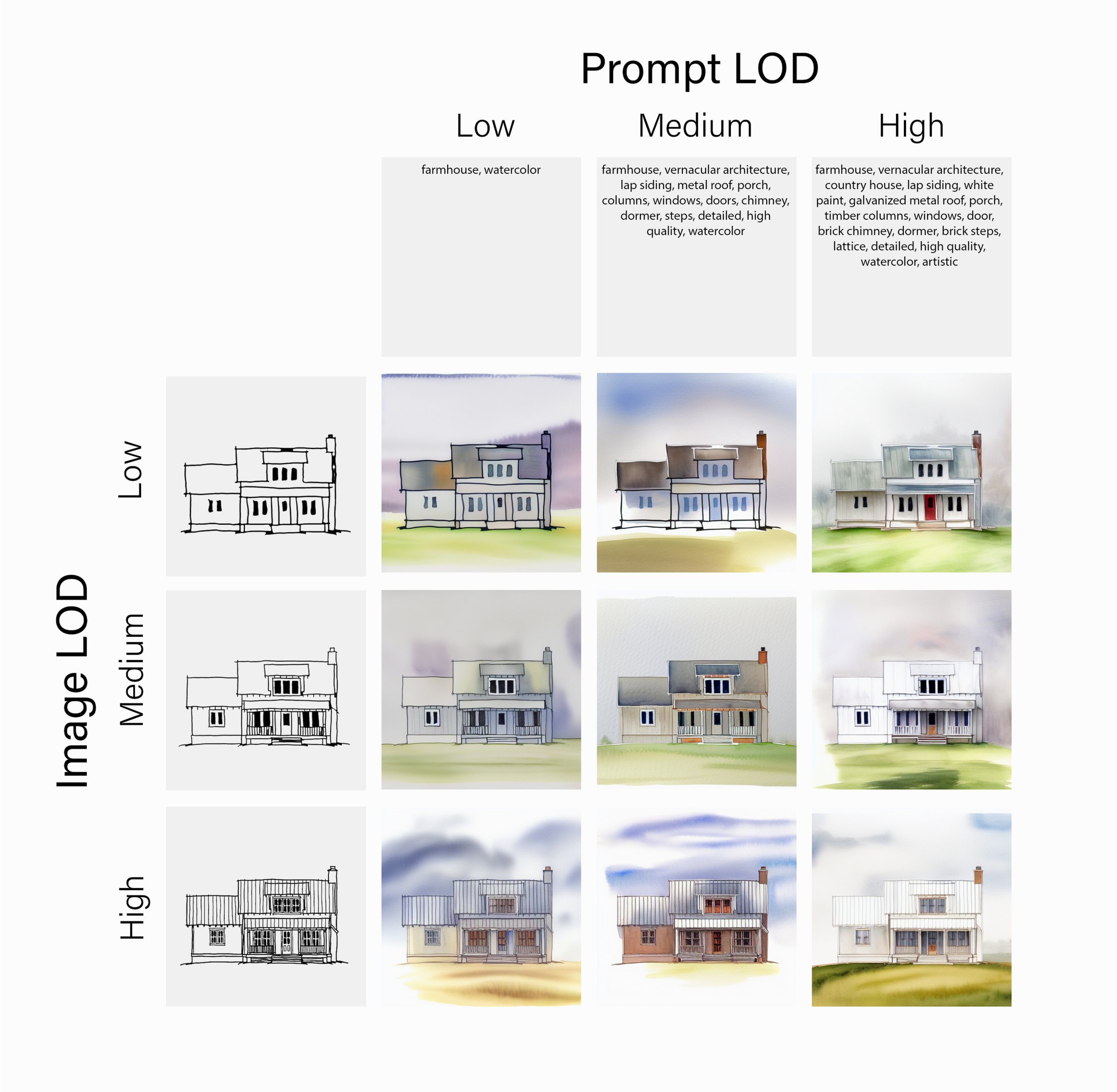
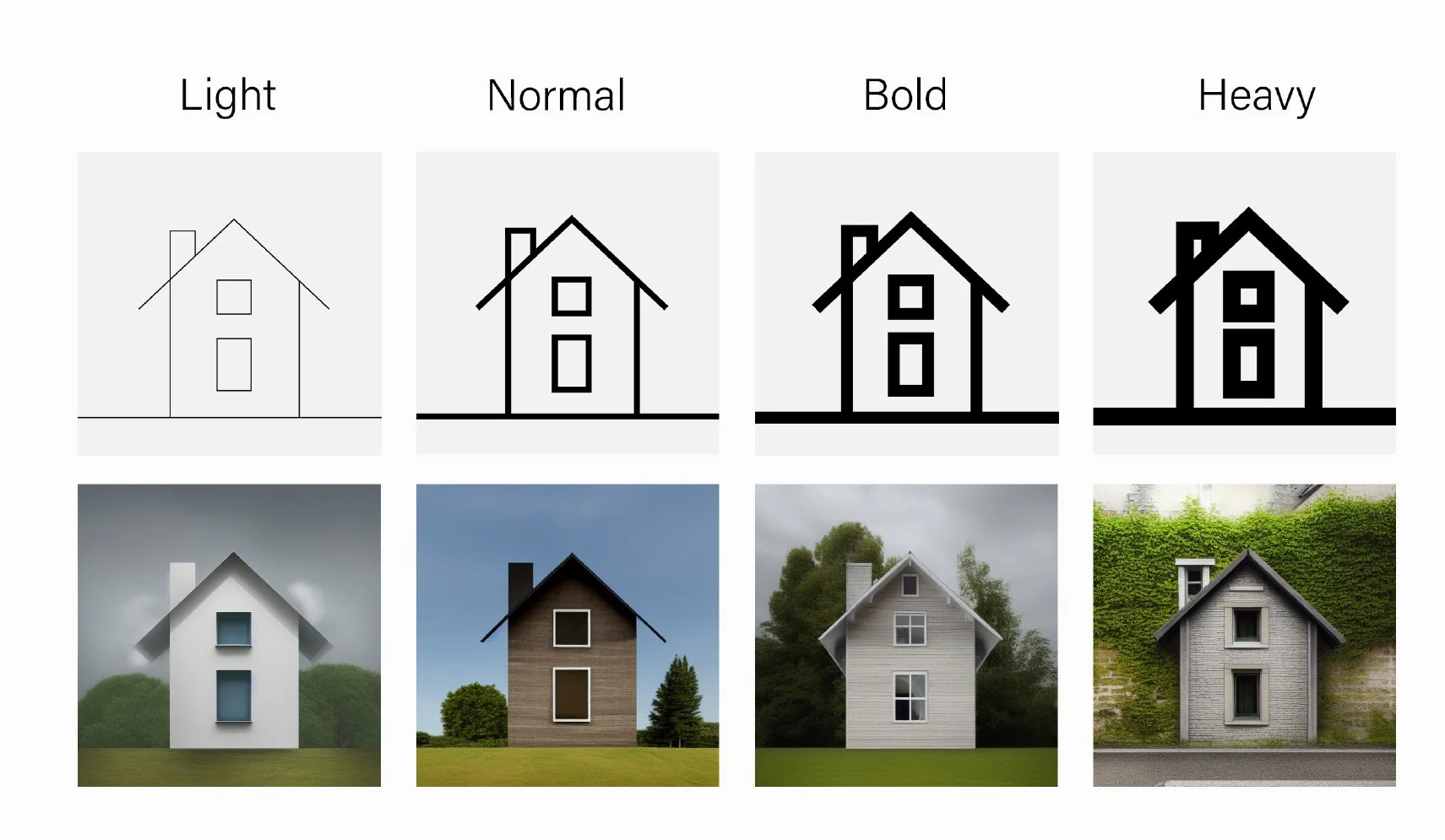
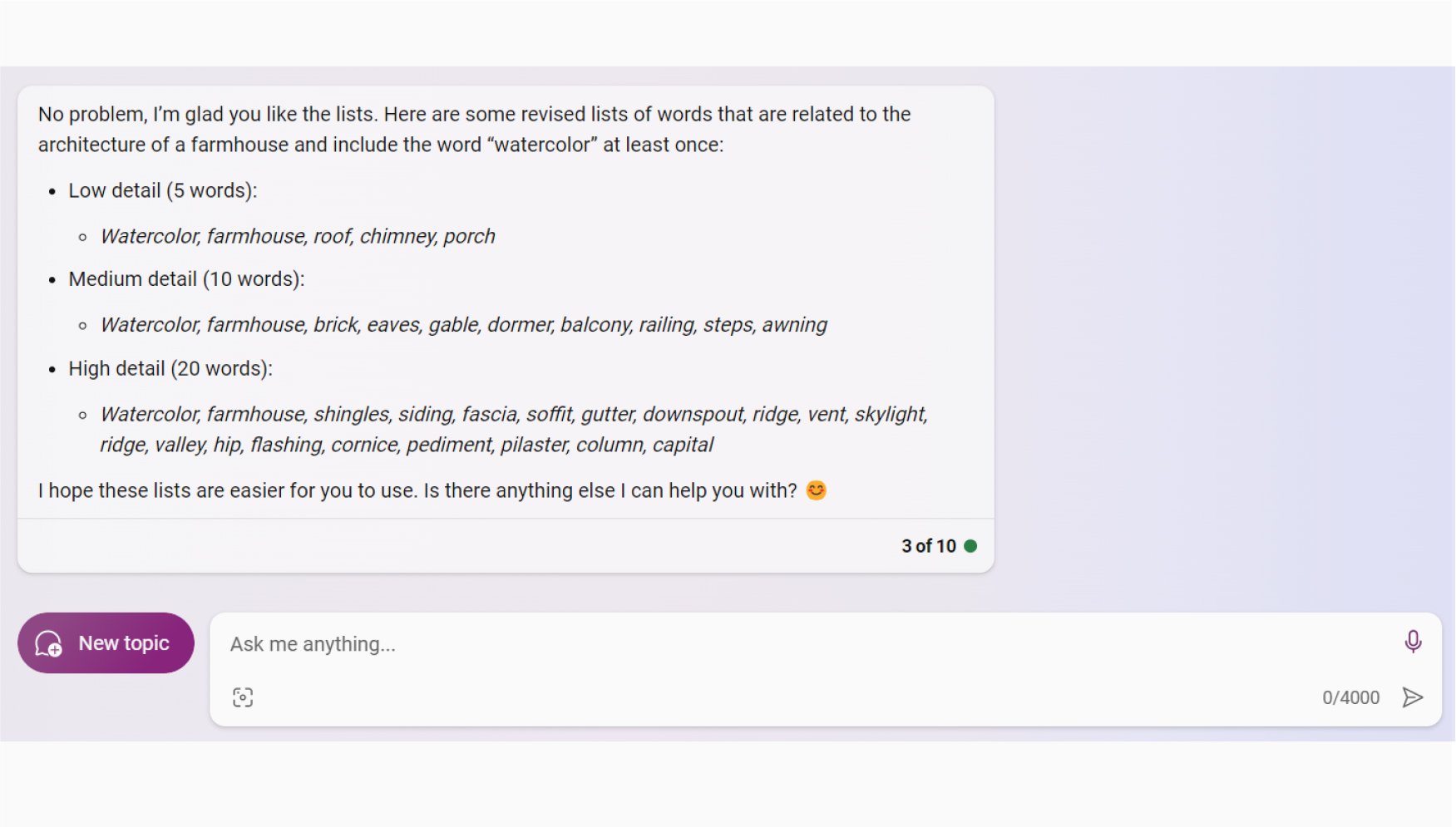

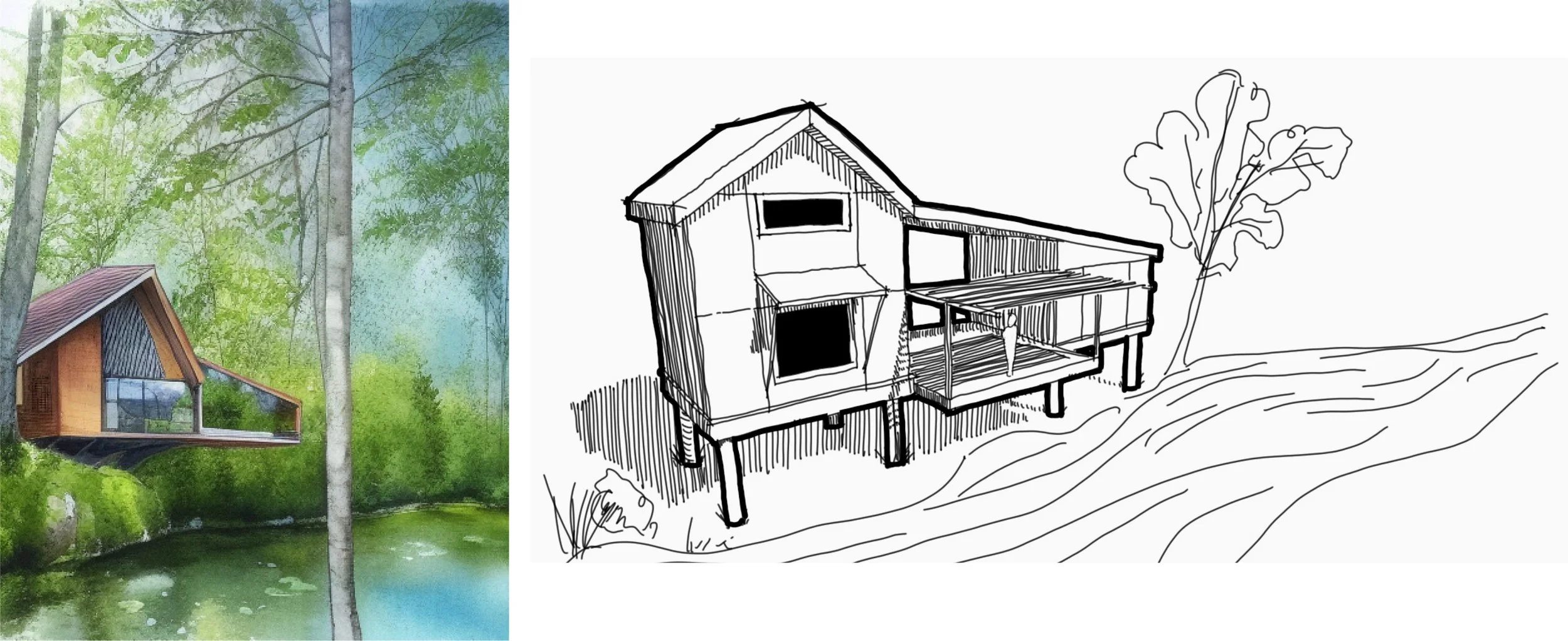
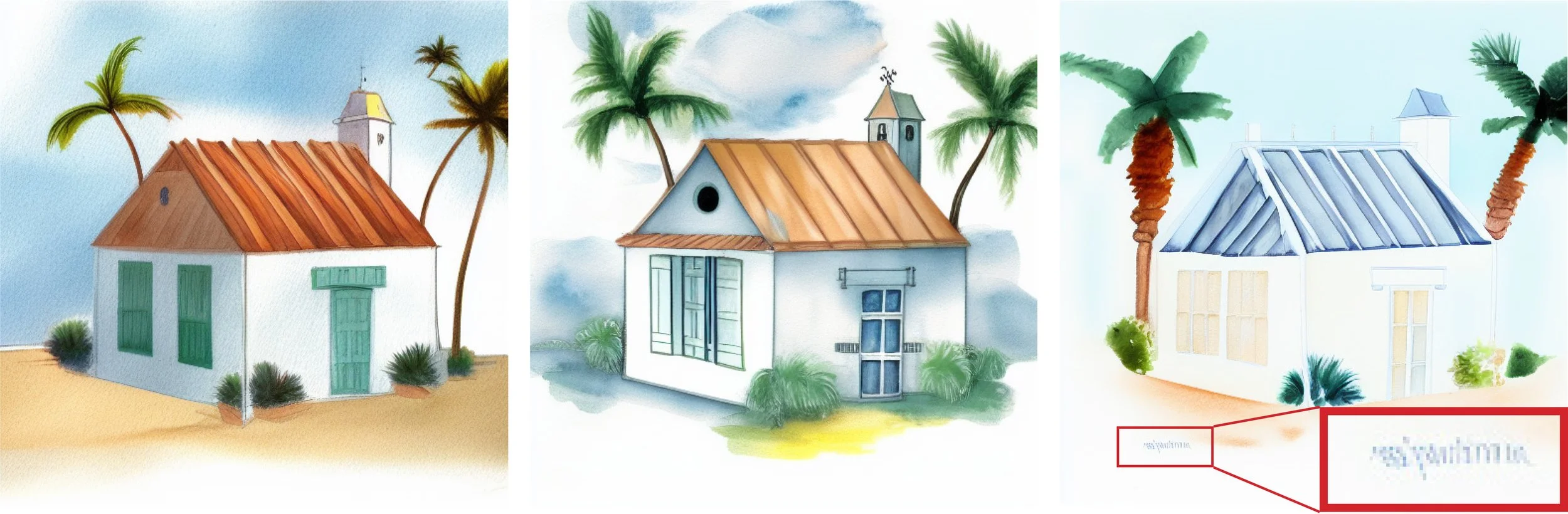
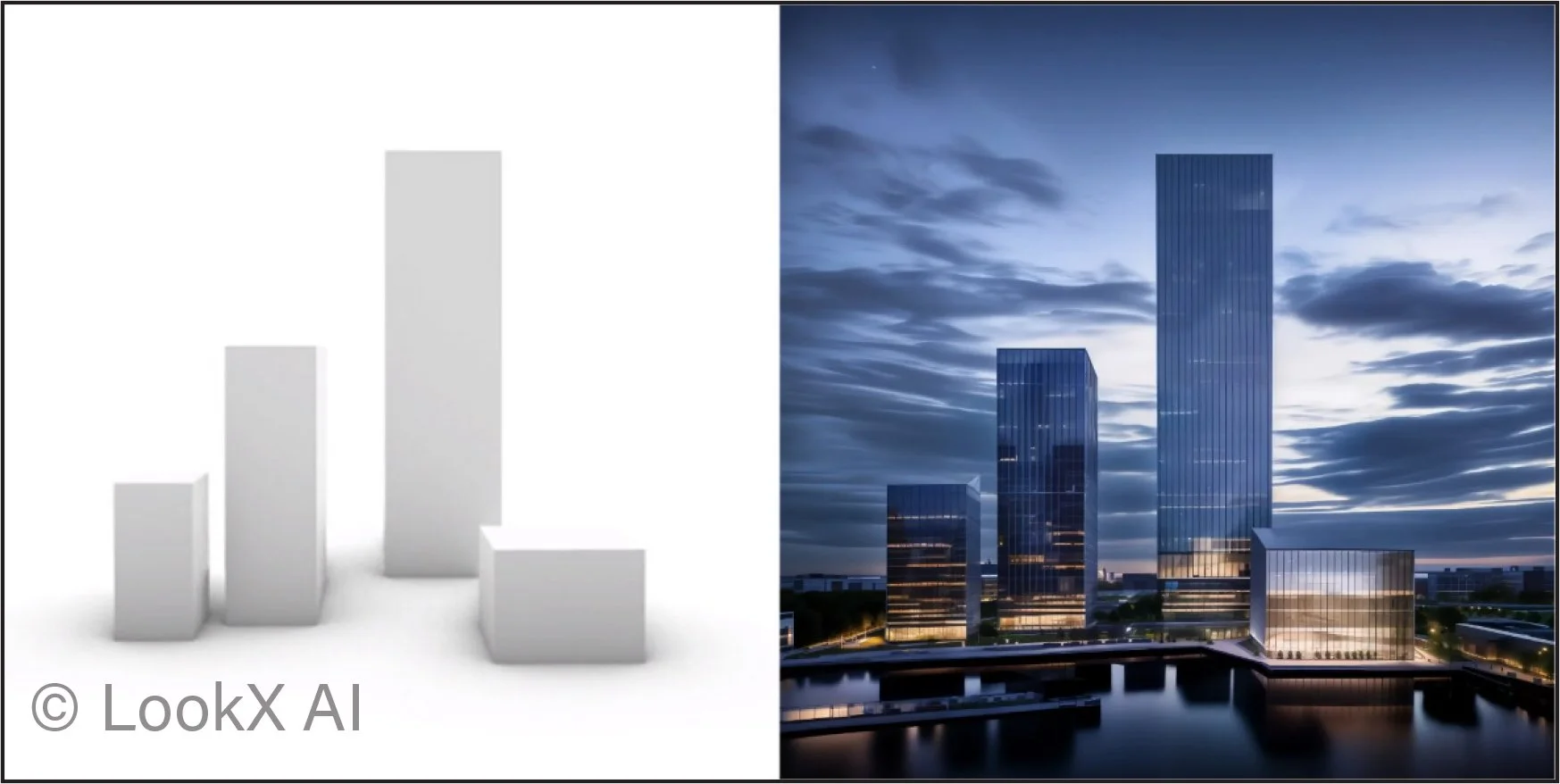